Machine Learning becomes Mainstream: How to increase your competitive advantage
First there was big data – extremely large data sets that made it possible to use data analytics to reveal patterns and trends, allowing businesses to improve customer relations and production efficiency. Then came fast data analytics – the application of big data analytics in real-time to help solve issues with customer relations, security, and other challenges before they became problems. Now, with machine learning, the concepts of big data and fast data analytics can be used in combination with Artificial Intelligence (AI) to avoid these problems and challenges in the first place.
So what is machine learning, and how can it help your business? Machine learning is a subset of AI that lets computers ‘learn’ without explicitly being programmed. Through machine learning, computers can develop the ability to learn through experience and search through data sets to detect patterns and trends. Instead of extracting that information for human comprehension and application, it will use it to adjust its own program actions.
How can #MachineLearning give a business an upper hand? #BigData #DataScience Click To Tweet
What does that mean for your business? Machine learning can be used across industries, including but not limited to healthcare, automotive, financial services, cloud service providers, and more. With machine learning, professionals and businesses in these industries can get improved performance in a number of areas, including:
- Image classification and detection
- Fraud detection
- Facial detection/recognition
- Image recognition/tagging
- Big data pattern detection
- Network intrusion detection
- Targeted ads
- Gaming
- Check processing
- Computer server monitoring
In their raw data, large and small data sets hide numerous patterns and insights. Machine learning gives businesses, organizations, and institutions the ability to discover trends and patterns faster than ever before. Practical applications include:
- Genome mapping
- Enhanced automobile safety
- Oil reserves exploration
Intel has worked relentlessly to develop libraries and reference architectures that not only enable machine learning but allow it to truly take flight and give businesses and organizations the competitive edge they need to succeed.
In fact, according to a recent study by Bain (1), companies that use machine learning and analytics are:
- Twice as likely to make data-driven decisions
- Five times as likely to make decisions faster than competitors
- Three times as likely to have faster execution on those decisions
- Twice as likely to have top-quartile financial results
In other words, predictive data analytics and machine learning are becoming necessities for businesses that wish to succeed in today’s market. The right machine learning strategy can put your business ahead of the competition, reduce your TCO, and give you the edge your business needs to succeed.
Background on Predictive Analytics and Machine Learning
You already know that machine learning is essentially a form of data analytics, but where did it come from and how has it evolved to become what it is today? In the past couple of decades, we have seen a rapid expansion and evolution of information technology. In 1995, data storage cost around $1000/GB; by 2014 that cost had plummeted to $0.03/GB (2). With access to larger and larger data sets, data scientists have made major advances in neural networks, which have led to better accuracy in modeling and analytics.
As we mentioned earlier, the combination of data and analytics opens up unique opportunities for businesses. Now that machine learning is entering the mainstream, the next step along the path is predictive analytics, which goes above and beyond previous analytics capabilities.
What's the path to #PredictiveAnalytics? #MachineLearning #BigData #DataScience Click To Tweet
The path to Predictive Analytics
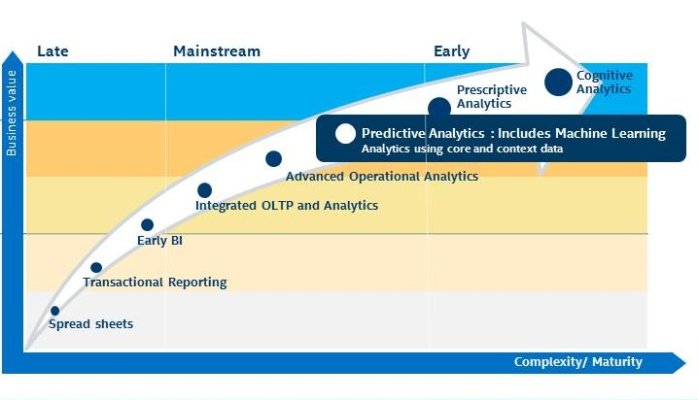
With Predictive Analytics, companies can see more than just ‘what happenned’ or ‘what will happen in the future’
Machine learning is a part of predictive analytics, and it is made up of deep learning and statistical/other machine learning. For deep learning, algorithms are applied that allow for multiple layers of learning more and more complex representations of data. For statistical/other machine learning, statistical algorithms and algorithms based on other techniques are applied to help machines estimate functions from learned examples.
Essentially, machine learning allows computers to train by building a mathematical model based on one or more data sets. Then those computers are scored when they may make predictions based on the available data. So when should you apply machine learning?
There are a number of times when applying machine learning can give you a competitive advantage. Some prominent examples include:
- When there is no available human expertise on a subject. Recent navigation to Pluto relied on machine learning, as there was no human expertise on this course.
- When humans cannot explain their abilities or expertise. How do you recognize someone’s voice? Speech recognition is a deep-seated skill, but there are so many factors in play that you cannot say why or how you recognize someone’s voice.
- When solutions change over time. Early in a rush-hour commute, the drive is clear. An hour later, there’s a wreck, the freeway comes to a standstill, and side streets become more congested as well. The best route to getting to work on time changes by the minute.
- When solutions vary from one case to another. Every medical case is different. Patients have allergies to medications, multiple symptoms, family histories of certain diseases, etc. Solutions must be found on an individual basis.
When should you apply #MachineLearning to improve your business? #BigData #DataScience Click To Tweet
These are just a few of the uses that you’ll find across industries and institutions for machine learning. Not only is the demand for machine learning growing, though, but there is now an evolving ecosystem of software dedicated to furthering machine learning and giving businesses and organizations the benefits of instantaneous, predictive analytics.
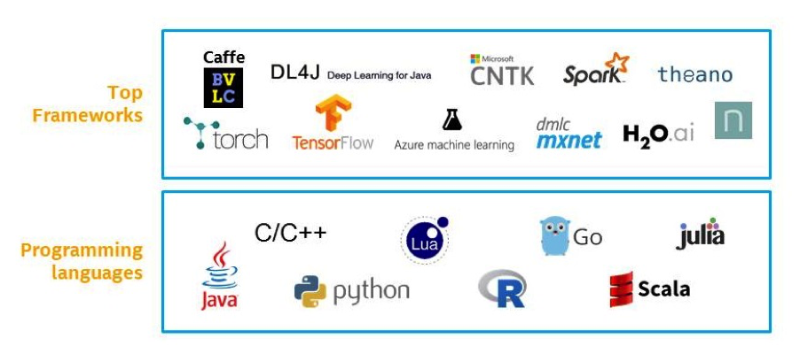
An evolving ecosystem of machine learning software (*)
In this ecosystem, Intel is the most widely deployed platform for the purposes of machine learning. Intel® Xeon® and Intel® Xeon Phi™ CPUs provide the most competitive and cost efficient performance for most machine learning frameworks.
What are the challenges to adoption of #MachineLearning? #BigData #Analytics #DataScience Click To Tweet
Challenges to adoption of Machine Learning
There are a few barriers to adoption of machine learning that businesses need to overcome to take advantage of predictive analytics. These include:
- Understanding how much data is necessary
- Adapting and using current data sets
- Hiring data scientists to create the best machine learning strategy for your business
- Understanding potential needs for new infrastructure vs. using your existing infrastructure
With the right machine learning strategy, the barriers to adoption are actually fairly low. And, when you consider the reduced TCO and increased efficiency throughout your business, you can see how the transition can pay for itself in very little time. As well, Intel is dedicated to establishing a developer and data science community to exchange thought leadership ideas across disciplines of advanced analytics. Through these articles and information exchanges, we hope to further help businesses and organizations understand the power of predictive analytics and machine learning.
What is your opinion and how do you apply data analytics and machine learning? Let us know what you think.
This article was co-authored with Nidhi Chappell, director of machine learning strategy at Intel Corporation.
Intel, the Intel logo, Intel Xeon, and Intel Xeon Phi are trademarks of Intel Corporation in the U.S. and/or other countries.
(*) Other names and brands may be claimed as the property of others. © Intel Corporation.
(1) http://www.bain.com/publications/articles/big_data_the_organizational_challenge.aspx (2) http://www.mkomo.com/cost-per-gigabyte-update